In my experience uncertainties come in two main types:
1. Where multiple solutions are possible but the best solution is unclear
Example: You may not know how best to store your data but there are many established methods available, so you just need to choose the most appropriate.
In these cases this is a lower order uncertainty. The uncertainty is not over whether it can be done, it’s about finding the most effective way of doing it. Treat these as key decision points. If there is no significant cost or outcome difference then these decisions should be left to the delivery team to resolve.
2. Where no solution is obvious
Example: You are attempting some new paradigm that requires interfacing with a system that is locked down and has never granted access before; there is no pattern established as to how this might work.
These types of uncertainties should be dealt with urgently. If this problem can’t be overcome then this could delay, halt or cause cost overruns as you work around it. You need to understand problems like these as quickly as possible. Quick, short experiments ought be run to understand the problem better until you have found at least one path forward.
Having addressed immediate uncertainties, inevitably more uncertainties will emerge. These subsequent problems tend to have a smaller impact and become more localised as the overall domain becomes better understood. The circles of uncertainty should keep closing in, from large problems to smaller and smaller ones.
Uncertainty around estimating time and cost of delivery – delivering on time
When there is so much uncertainty around delivery, managing expectations on cost and timings is not straightforward.
Emergent work is hard to estimate accurately as little experience exists to use as a baseline. The only reasonably accurate estimates in a program tend to be in those parts that are founded on practical experience, things that have been done before.
Successful projects, however, do tend to find ways to predict the effort. They work effectively with sponsors and stakeholders and land their outcomes on expectation.
Successful programs show a preference for continuous delivery of value in order to offset the ambiguity around cost. This swaps the problem from being an estimation problem to being a prioritisation problem. As value gets delivered, decisions can be made at regular intervals to continue the investment to garner further value.
There will however be projects where continual delivery is less practical – where the value isn’t realised until all the parts are assembled – despite all the warnings from textbooks. Assuredly, the program will still be asked when? And, how much?
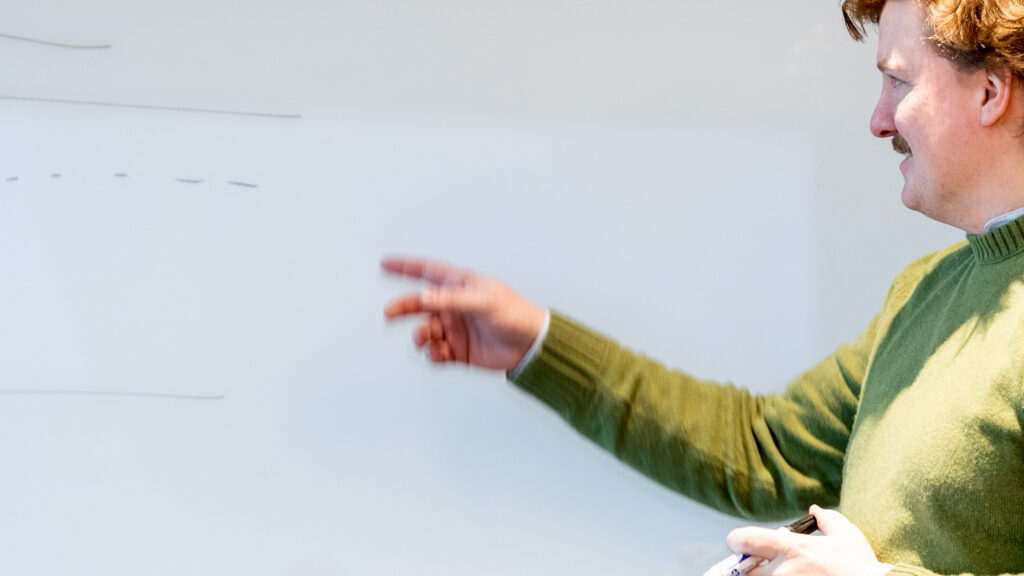
In this case, there are two strategies I’ve seen work:
- Rather than estimate everything at once, work backwards from a target date
Example: If the outcome is to land in a year what needs to be true by mid-year, and from there, what needs to be true by end of Q1? At this point, ask delivery teams to quote on achieving that more digestible effort. That will result in a target date and an idea of what the first quarter will cost, both of which you can use to inform stakeholders and sponsors.
- If you have compartmentalised the program well, then quite a few work streams should be less uncertain
On those streams take a rough estimate from the senior engineers (or the ones who have done this before and understand the platform best) and use this to baseline an indicative cost.
For the other streams, ask the most experienced engineers for a blink estimate, i.e. a rough guess at how hard it is. And just use that. It will tend to be more accurate and a lot less effort than many other methods I’ve seen over the years.
When communicating with stakeholders and sponsors, quote the uncertainty and as uncertainties get resolved refine the estimates and report the changed expectations, particularly if they breach some ceiling. This gives them control over cost management.